Welcome!
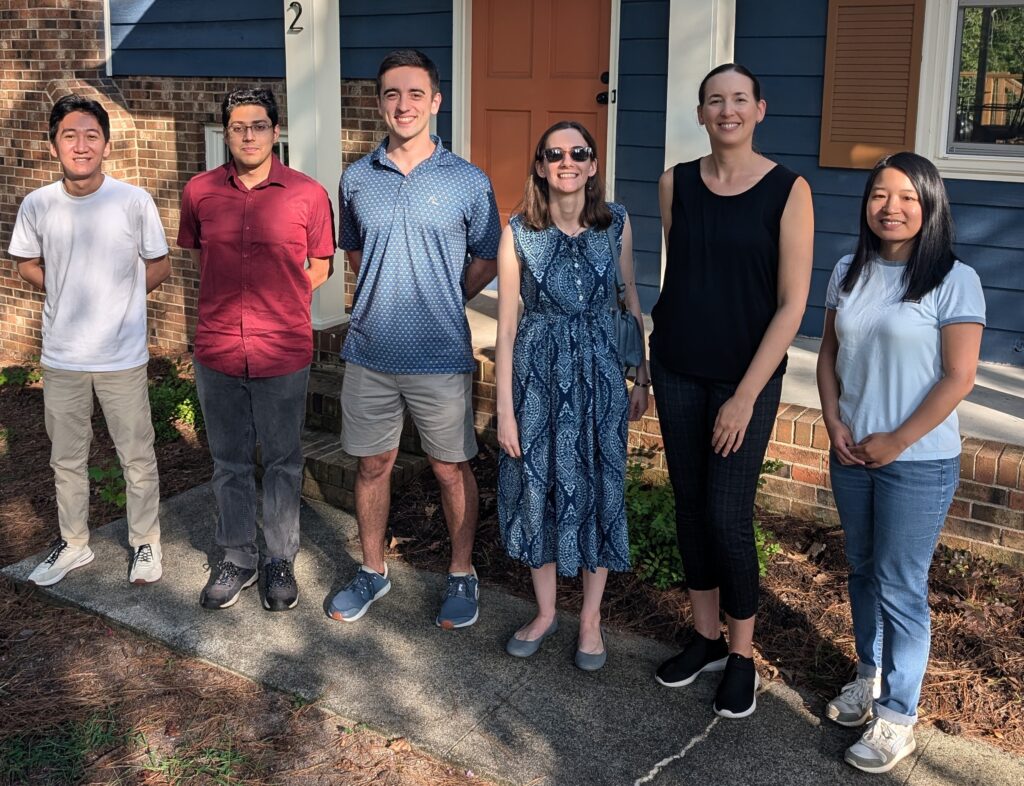
We are interested in developing numerical methods for nonlinear and high-dimensional data analysis. Our focus lies on algorithms that preserve geometric structures, and our work includes optimal transport problems, classification tasks in machine learning, linear and nonlinear approximation, and applications in biology and cancer research.
People
Caroline Moosmueller
Amartya Banerjee
Kaitlyn Hohmeier
Aaron Jacobson
Chuxiangbo Wang
Research
Computational optimal transport
Approximation theory
Biology and cancer research
- SIAM news on Caroline’s talk at MDS 24Caroline’s talk “Trajectory inference in Wasserstein space” at SIAM MDS 2024 in Atlanta was featured in SIAM news! Overall, we (Amartya, Caroline, Shiying) had a great time at SIAM MDS, presenting two talks, two posters, and organizing a mini-symposium.
- UNC Data Science Day & TriCAMS 2024 – poster prizes!Aaron Jacobson Amartya Banerjee Kaitlyn Hohmeier Chuxiangbo Wang Great turnout of the Geometric Data Analysis group at UNC’s Data Science Day on Sep 26, 2024 and at TriCAMS 2024 on Oct 26 – 27, 2024. Two poster prizes at the Data Science day and one poster prize at TriCAMS were won! Aaron presented his poster… Continue reading UNC Data Science Day & TriCAMS 2024 – poster prizes!
- Paper on Approximation properties of slice-matching operatorsShiying’s and Caroline’s paper “Approximation properties of slice-matching operators” is available on the arXiv. In this paper we iterative slicing-and-matching schemes, in particular, the approximation power of one step of such schemes. We show an invariance and an equivariance property with respect to global rigid transformations, approximation of the target measure, and derive a connection… Continue reading Paper on Approximation properties of slice-matching operators
- Paper on stochastic slicing and matchingA preprint of Shiying’s and Caroline’s paper “Measure transfer via stochastic slicing and matching” is available on the arXiv. In this paper we discuss an iterative slicing-and-matching procedure which can be used for measure approximation. This scheme has first been introduced by PitiĆ© et. al.; we show a convergence proof of a stochastic version.
- Data science seed grantCaroline, together with Shahar Kovalsky, Martin Styner, and Guorong Wu received a seed grant from the School of Data Science and Society at UNC. Our award on “Spatio-temporal analysis of brain functional connectome” will run until June 2024. Official announcement Abstract: We will develop mathematical models and algorithms for the analysis and statistical characterization of… Continue reading Data science seed grant