Highlighted Projects
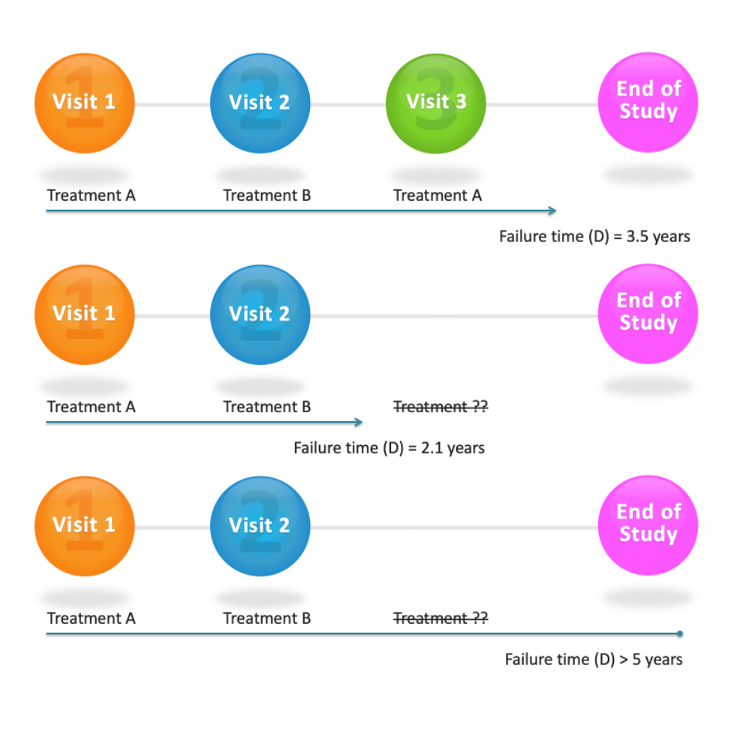
Dynamic treatment regimes for survival outcomes
By Hunyong Cho
With a goal of maximizing cancer patients’ survival time, deriving an optimal dynamic treatment regime (DTR) requires careful consideration of censoring. To this end, we developed a flexible Q-learning framework that uses random forests.
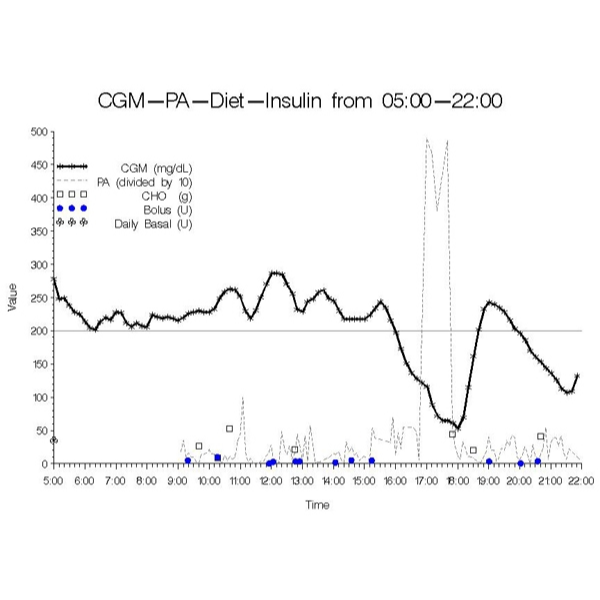
V-Learning and mHealth methods for management of type 1 diabetes
By Anna Kahkoska
In contrast to standard settings for dynamic treatment regime (DTR) estimation, mobile health (mHealth) applications provide a large number of observations per individual at a very fine granularity. One such example is type 1 diabetes, where DTRs can have significant implications on the day-to-day management of blood glucose levels. We have developed a reinforcement learning method, V-learning, which attempts to alleviate the difficulties of mHealth data by estimating an optimal policy without posing modeling assumptions on the data generating process.
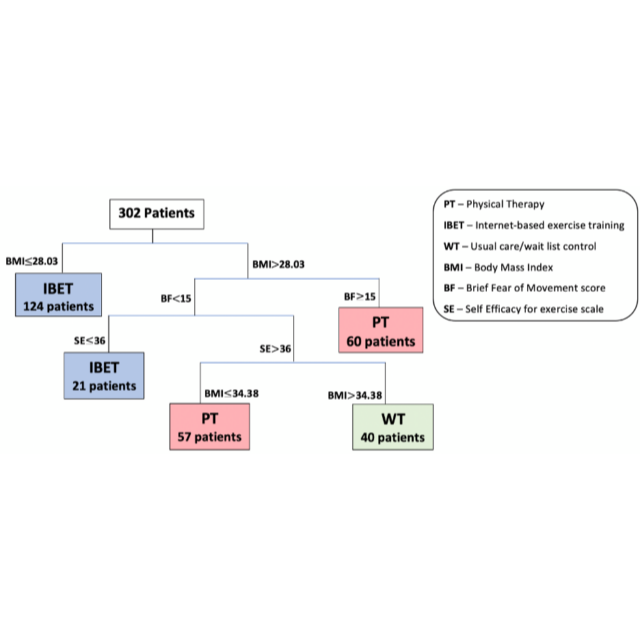
Precision medicine for knee osteoarthritis
By Siyeon Kim
The precision medicine approach has been actively applied to arthritis studies. We have developed a new tree-based method, Random Forest Informed Tree-based Learning, to identify underlying patient characteristics that impacted different improvements in patient outcome while incorporating the advantages of random forests.