
- This event has passed.
FRG Informal Talk Series: Ethan Wood and Yang Yang
November 30, 2023 @ 2:00 pm - 3:00 pm
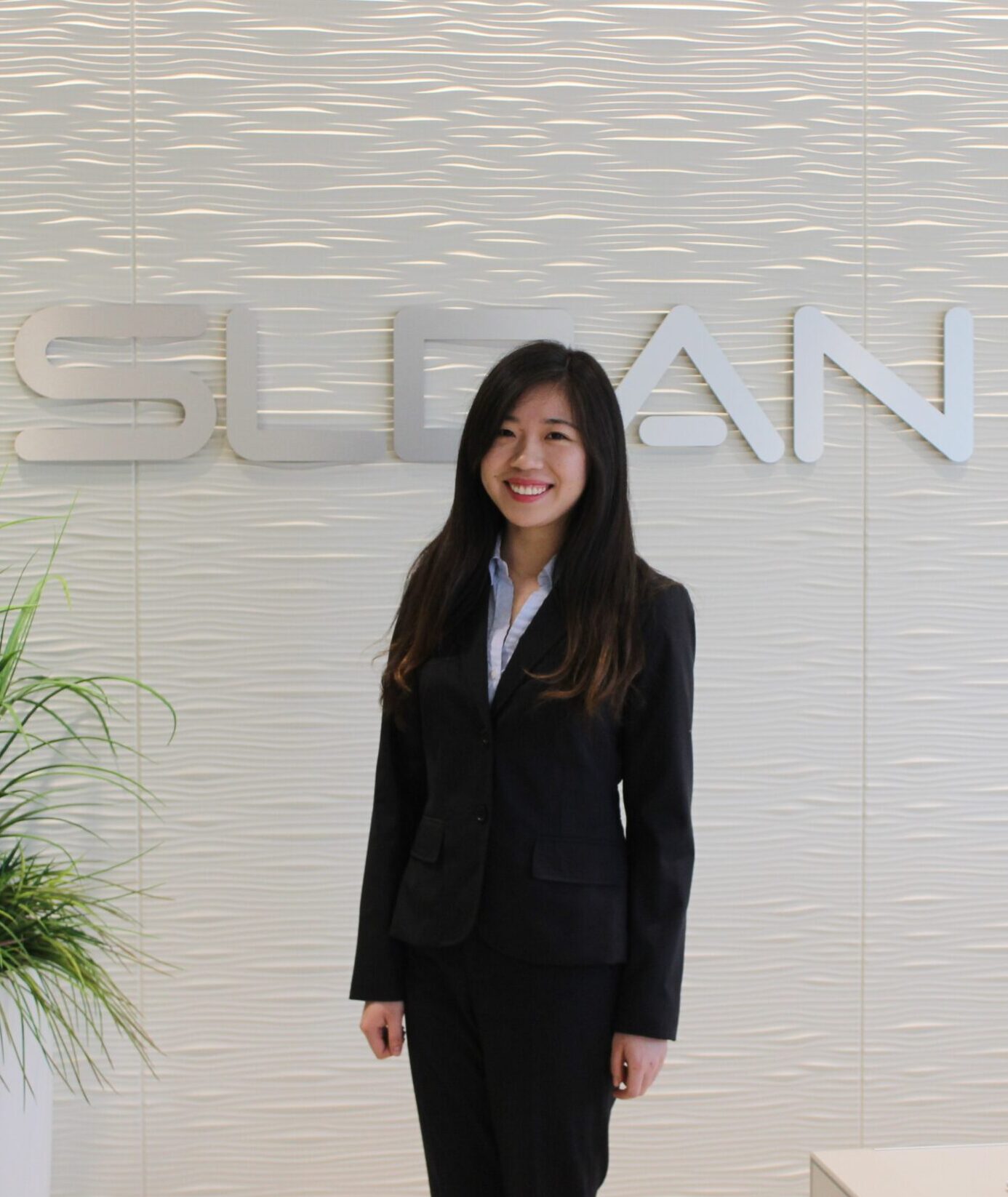
2:00-2:20pm Lighting talk by Ethan Wood
Title: Analyzing Arsenic and Water Quality with MaxBET
Abstract: The United States Environmental Protection Agency published a paper and a dataset focusing on measured levels of arsenic in community water systems (CWS) per county and instances of cancer in those respective counties. By applying MaxBET to the dataset the EPA published, I found non-linear relationships between both weighted and unweighted levels of arsenic, and water quality. Rather than there existing a simple linear relationship between water quality and measured arsenic, there exists a parabolic relationship between weighted arsenic and water quality and a horizontal parabolic relationship between unweighted arsenic and water quality. The non-linearity of the weighted arsenic might be explained by its formula, which depends on the population of the county, but the unweighted arsenic seems to be slightly more complicated. Viewing clusters of states and regions in the US appears to provide a possible explanation for non-linearity, due to arsenic being geogenic in origin. Further research will be continued to see how this geogenic quality of arsenic applies to instances of cancer in each county.
2:20-3:00pm Informal talk by Yang Yang
Title: Conditional Independent Tests with DeepBET
Abstract: Conditional independence (CI) serves as a fundamental cornerstone in statistics, machine learning, and artificial intelligence. This project focuses on the assessment of conditional independence between two random univariate variables, X and Y, given a set of high-dimensional confounding variables Z. The dimensionality of Z poses a challenge for many existing tests, leading to either inflated type-I errors or insufficient power in detecting alternatives.
To address this issue, we leverage the Deep Neural Network (DNN)’s ability to handle complex, high-dimensional data while circumventing the curse of dimensionality. We propose the utilization of a DNN model to estimate the conditional means of X and Y given Z using part of the data and obtain predicted errors using the other part of the data. We then apply novel binary expansion statistics as our test metrics to predicted errors for dependence detection. Furthermore, we implement the multiple split method to enhance power, utilizing the entirety of the sample while minimizing randomness. Our preliminary results show that the proposed method adeptly controls type I error control and exhibits a significant capacity to detect alternatives, making it a robust approach for testing conditional independence in the presence of high-dimensional confounding variables.
The talks will be on Zoom: https://unc.zoom.us/j/93583000576. They will not be recorded.
Leave a Reply
You must be logged in to post a comment.